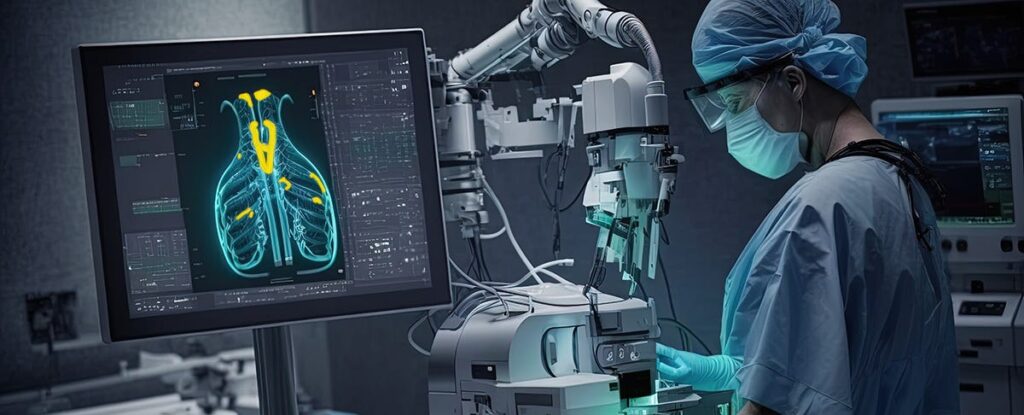
In the evolving landscape of healthcare, data is not just a byproduct of medical processes—it is the bedrock of groundbreaking innovations that are reshaping patient care. With the increasing integration of artificial intelligence (AI) in medicine, the accuracy and reliability of AI predictions are contingent on the quality of data that feeds these models. Medical data annotation, the process of labeling and structuring healthcare data to make it comprehensible for machine learning algorithms, plays a critical role in enabling these transformative advancements.
What is Medical Data Annotation?
Medical data annotation involves categorizing raw healthcare data into understandable formats, enabling machine learning models to process and extract insights. This process typically includes the annotation of medical images, electronic health records (EHR), pathology reports, and more. For instance, annotating radiological images, such as CT scans and MRIs, helps AI systems identify and diagnose medical conditions with a level of precision that can assist medical professionals in providing accurate treatments.
Types of Medical Data That Require Annotation
- Medical Images: Images such as X-rays, MRIs, and CT scans are crucial for diagnosing conditions like cancer, neurological disorders, and fractures.
- EHR (Electronic Health Records): Textual data from patient records, including diagnostic results, treatment plans, and medical histories.
- Pathology Reports: Annotations in pathology reports help in training AI to identify patterns and correlations in disease progression.
- Genetic Data: Genomic annotations support personalized medicine by helping AI systems create tailored treatment plans based on an individual’s genetic makeup.
Key Applications of Medical Data Annotation
The integration of AI in healthcare is fueled by accurate data annotation, offering applications across various healthcare domains. These applications enhance operational efficiency, improve patient outcomes, and contribute to the advancement of medical technology.
1. Medical Imaging Analysis
Medical imaging is one of the most vital applications of medical data annotation. Annotated images enable AI models to detect conditions such as tumors, fractures, and other abnormalities. By training AI to recognize these patterns, radiologists are equipped with powerful diagnostic tools that provide enhanced accuracy and reduce the chances of human error.
2. Natural Language Processing (NLP) in Healthcare
NLP algorithms, when trained on annotated healthcare texts, can identify key information such as symptoms, diagnoses, and medications within unstructured data. This allows healthcare providers to quickly retrieve actionable insights from massive datasets like doctor’s notes, discharge summaries, and medical literature.
3. Drug Discovery and Research
Medical data annotation accelerates drug discovery by labeling molecular and clinical trial data. AI models can predict drug efficacy, potential side effects, and patient reactions, drastically reducing the time and cost involved in bringing new drugs to market. Annotated datasets are essential for refining these AI models, ultimately contributing to more effective therapies and treatments.
4. Robotic Surgery and Precision Medicine
AI-powered robotic systems require annotated data to deliver precision during surgery. For instance, annotated anatomical images guide robotic surgery systems to make accurate, real-time decisions. Similarly, genetic data annotations enable AI to recommend personalized treatment options based on an individual’s genetic profile.
5. Disease Outbreak Monitoring
During global health crises, AI systems use annotated epidemiological data to predict the spread of diseases, allowing governments and healthcare organizations to respond swiftly and effectively. Annotated datasets help AI models identify disease hotspots, track infection rates, and optimize resource allocation.
Challenges in Medical Data Annotation
Despite the immense potential of medical data annotation, there are several challenges that need to be addressed to ensure the success of AI-powered healthcare systems.
1. Data Privacy and Compliance
Medical data is highly sensitive, and its annotation must adhere to strict privacy regulations such as HIPAA and GDPR. Ensuring that annotation practices comply with these laws is crucial to maintaining patient confidentiality and avoiding legal repercussions.
2. Domain Expertise Requirement
Accurate medical data annotation requires an in-depth understanding of medical terminology and practices. Annotators must possess domain expertise, often necessitating collaboration with healthcare professionals like radiologists, pathologists, and clinicians. This ensures that the data annotations are contextually accurate and meaningful.
3. High-Quality Data Needs
For AI models to produce reliable predictions, the data used for training must be of the highest quality. Any inconsistencies or errors in annotations could lead to flawed AI predictions, which could have serious consequences in a medical setting. Ensuring data consistency and accuracy is paramount.
4. Time and Cost Constraints
Medical data annotation is often a time-consuming and resource-intensive process. The labor involved in labeling and structuring vast amounts of data can lead to significant costs. Additionally, large-scale annotation projects require substantial workforce management to meet deadlines without compromising quality.
Best Practices for Effective Medical Data Annotation
Adopting the right strategies can mitigate challenges and ensure the success of medical data annotation projects. The following best practices provide a framework for producing high-quality annotated data while addressing the complexities of the healthcare sector.
1. Involve Medical Experts
Incorporating healthcare professionals in the annotation process ensures the accuracy and reliability of the labeled data. Their expertise in interpreting complex medical data helps avoid misinterpretations and ensures the contextual relevance of annotations.
2. Utilize Advanced Annotation Tools
AI-assisted annotation tools can streamline the data labeling process. These tools automate tasks such as image segmentation, enabling faster and more consistent annotations. Advanced tools also help reduce human error and increase the efficiency of large-scale projects.
3. Establish Clear Annotation Guidelines
To ensure consistency, it is essential to establish clear and detailed annotation guidelines. Annotators should receive explicit instructions on how to label various types of data, with examples to help maintain uniformity and minimize mistakes.
4. Implement Robust Quality Assurance (QA) Processes
Regular quality checks are vital to ensure the accuracy of annotated data. QA processes can include peer reviews, cross-validation, and periodic audits by domain experts. By identifying and rectifying errors early in the annotation process, organizations can prevent issues from affecting AI model training.
5. Ensure Data Security
Data security is a top priority in healthcare, and medical data annotation processes must adhere to stringent security protocols. Ensuring that all data is handled in compliance with privacy regulations and using secure platforms for annotation helps safeguard sensitive patient information.
The Future of Medical Data Annotation
As AI continues to shape the future of healthcare, the importance of medical data annotation will only grow. Innovations in technologies such as federated learning, blockchain, and advanced machine learning techniques are expected to address existing challenges such as scalability, data privacy, and annotation speed. These advancements will further accelerate the use of AI in medical applications, leading to faster diagnoses, more personalized treatments, and improved patient outcomes.
The increasing reliance on annotated medical data will drive innovations in both AI algorithms and the tools used to facilitate annotation. Future technologies will enable more efficient and accurate annotation workflows, reducing the time and cost involved in training AI models.
Conclusion: The Critical Role of Medical Data Annotation in Healthcare
Medical data annotation is the cornerstone of AI-driven healthcare innovations. From enhancing diagnostic accuracy to enabling personalized treatment plans and accelerating drug discovery, the applications of medical data annotation are vast and transformative. However, the process is not without its challenges, including data privacy concerns, the need for domain expertise, and the time-intensive nature of annotation tasks.
By adopting best practices such as involving medical professionals, utilizing advanced tools, and ensuring data security, healthcare organizations can overcome these challenges and unlock the full potential of AI in healthcare. As the industry continues to embrace artificial intelligence, the role of medical data annotation will only become more critical in advancing the future of medicine and improving patient care.